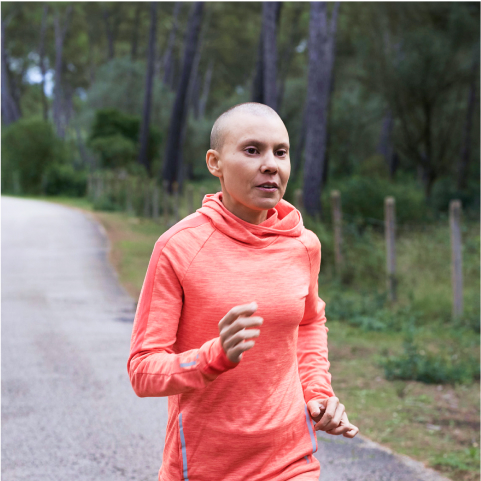
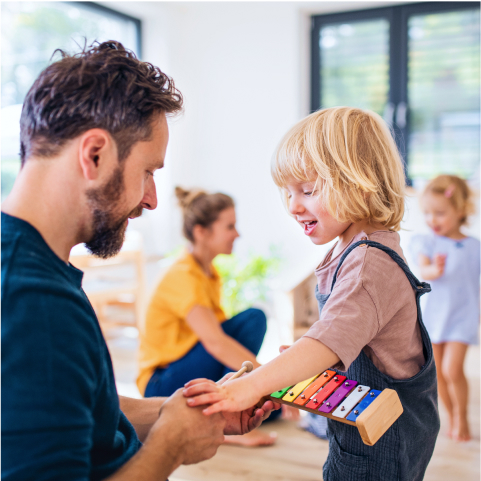
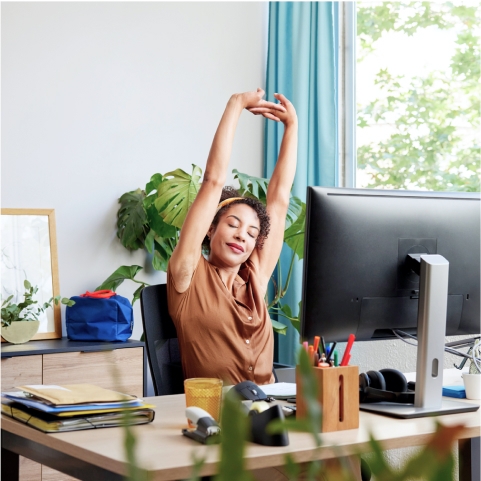
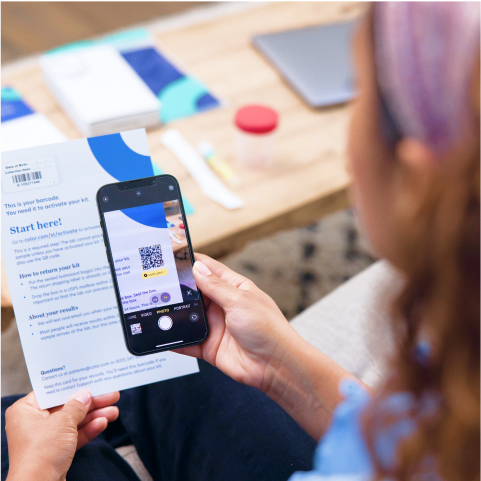
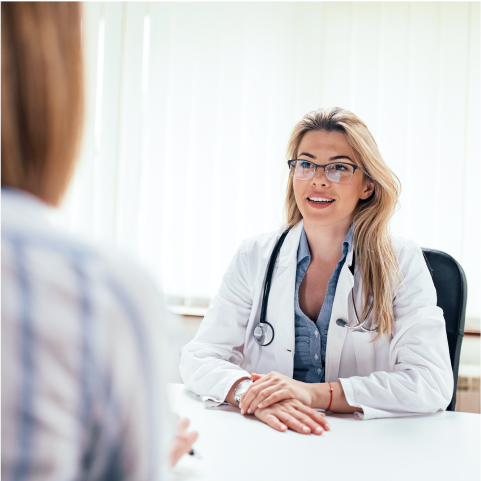
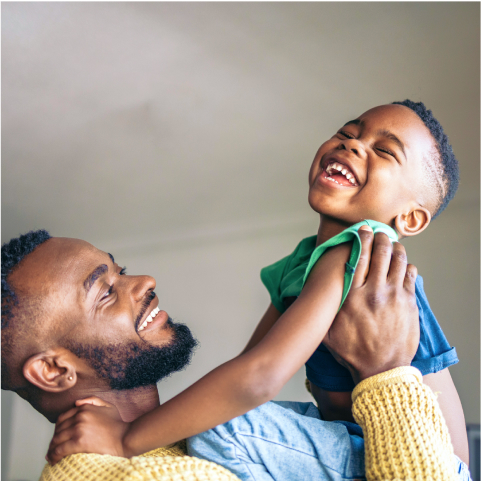
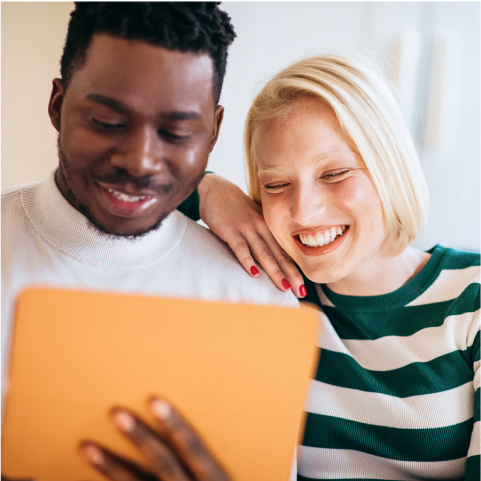
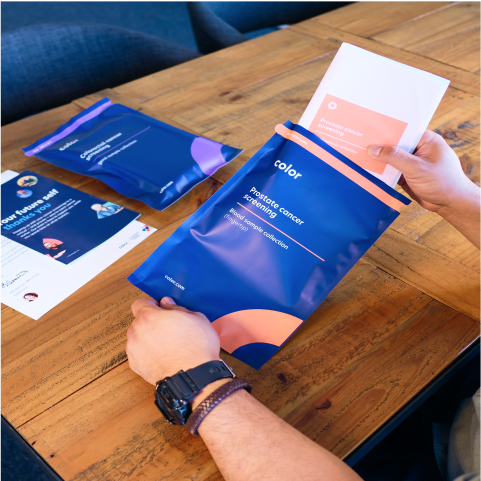
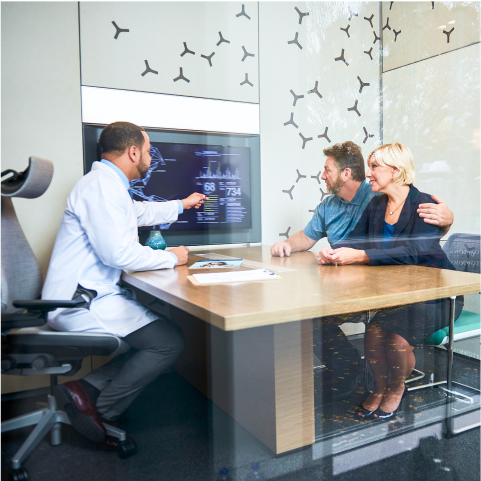
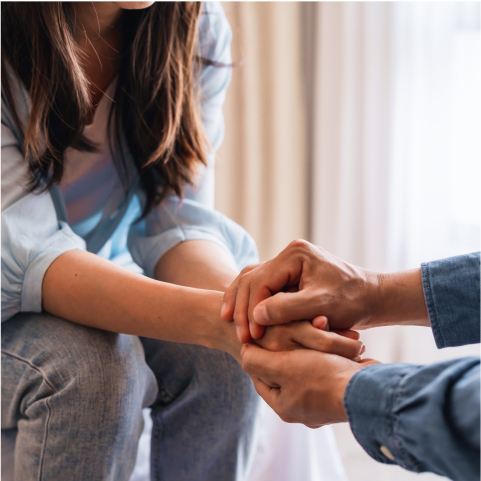
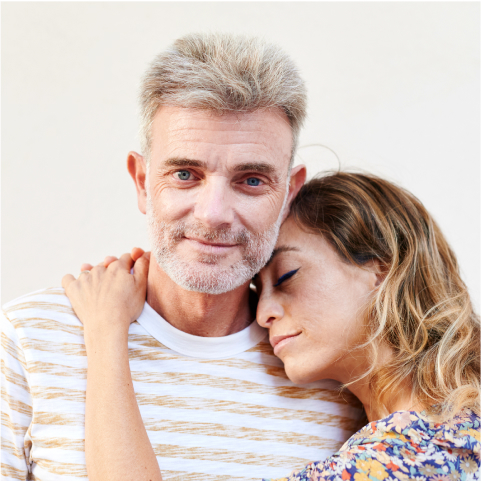
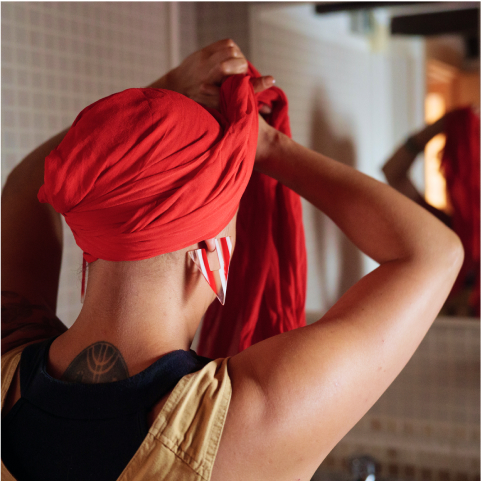
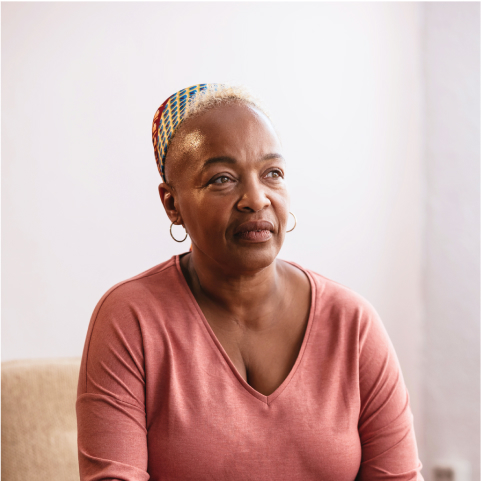
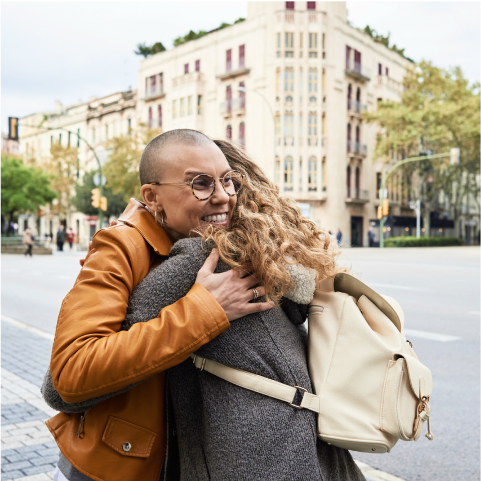
Designed for people.
Built for populations.
Our story
Today, Color works with employers, unions, health plans, and governments to take control of cancer through a comprehensive cancer program. But we’ve grown and evolved a lot since our founding in 2013—establishing a 50-state medical practice, serving over 7 million patients in our time, innovating on new and accessible sample collection for screening, and expanding our clinical and support teams.
No year has looked the same, but our north star has remained consistent for over a decade: providing accessible, convenient, easy-to-use healthcare services in critical health areas—cancer, infectious disease, and cardiometabolic disease—where barriers to access persist, despite available and affordable technology.
Read more about the major milestones in Color’s history below.
Since our beginning, we’ve been committed to improving access to essential healthcare.
Color Health launches its first product to help make healthcare more affordable, accessible, and actionable.
In 2015, Color launched with a new clinical genetic testing model to help patients understand and take action on their personal risk of cancer. Our first product tested for BRCA1, BRCA2, and 17 other genes that increase an individual’s cancer risk. At the time, genetic tests for ovarian and breast cancer risk typically cost several thousand dollars, and access was often gated through long wait times for genetic counseling appointments at centers of excellence. Color was the first to deliver comprehensive genetic testing—including genetic counseling, at-home sample collection, and easy-to-understand reports—for less than a tenth of the cost. This affordable model has helped hundreds of thousands of individuals take action on their genetic risk.
Learn how Color worked with the Teamsters Health and Welfare Fund of Philadelphia and Vicinity to change how employees engaged with preventive care by better understanding their risk of cancer and other genetic diseases.
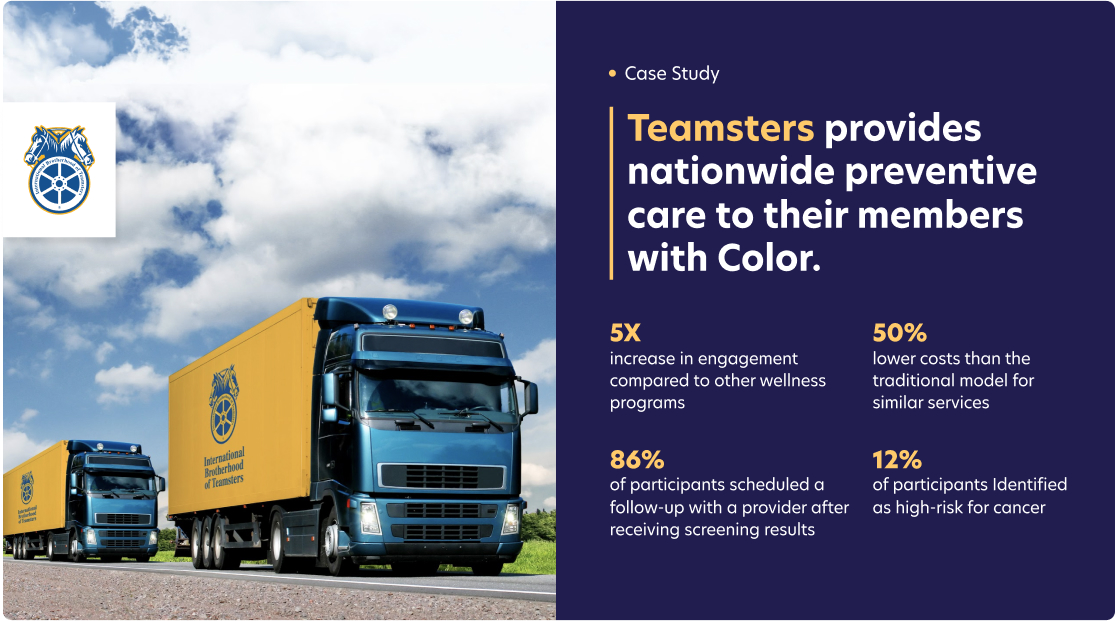
Color begins a long history of partnering with leaders in research and precision medicine, including the National Institutes of Health.
In 2018, the National Institutes of Health (NIH) awarded funding to Color, in partnership with the Broad Institute of MIT and Harvard, to establish one of three genome centers for its historic All of Us Research Program, a one million-participant program aimed at helping researchers better understand long-term health outcomes across the U.S. Color was initially selected to analyze and report genomic data for a set of 59 genes that cause conditions such as breast and ovarian cancer, hypercholesterolemia, Lynch syndrome, and more. Since then, Color has become the genetic counseling resource for all 1 million participants, the single home for test reporting, and the provider of ongoing participant engagement.
Since launching, the program has gathered data from hundreds of thousands of participants, over 80% of whom come from populations that have been historically underrepresented in clinical research. Our work with the NIH is the largest of our major research collaborations but not the last. We have since gone on to work with research institutions such as the Mayo Clinic, the Fred Hutchinson Cancer Research Center, and the University of Chicago and many local, state, and Federal governments.
Learn about an important NIH and Color partnership milestone—processing 100,000 genetic results for the All of Us Program—below.
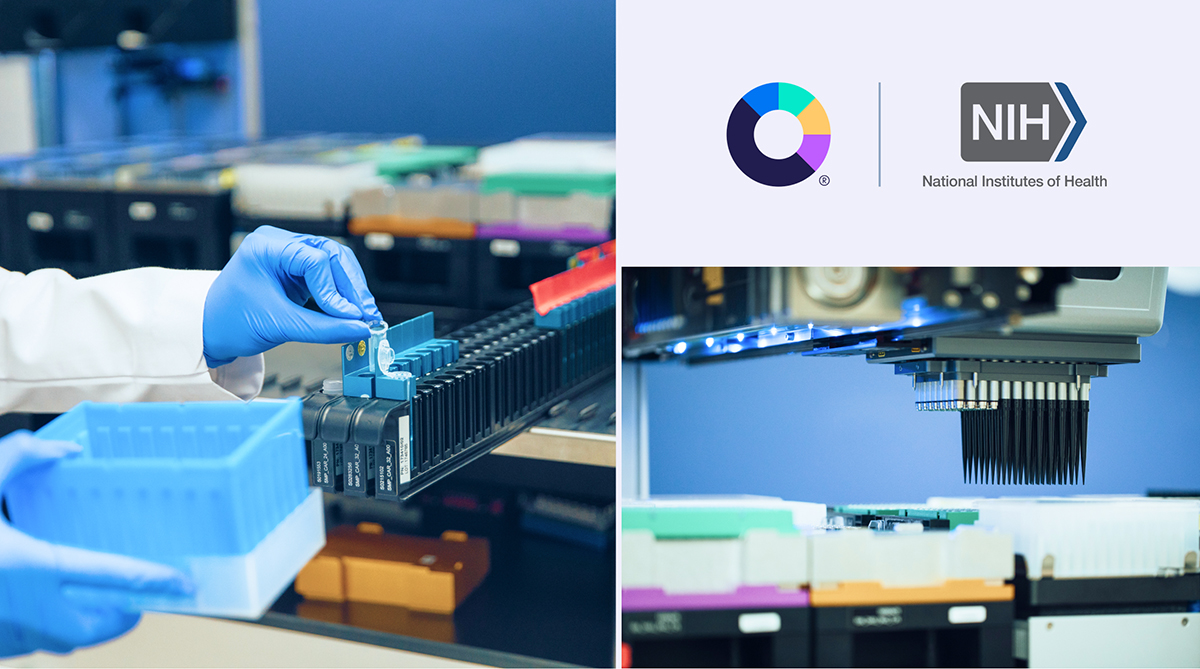
Color mobilizes to change access to critical COVID-19 testing, vaccination, and treatment services.
On March 16th, 2020, the first stay-at-home orders were issued in the San Francisco Bay Area. Exactly two weeks later, Color had built a CLIA-certified COVID-19 testing lab and digital platform. One week after that, Color and the City and County of San Francisco launched one of the first high-capacity, public COVID testing sites.
In the months and years to come, Color supported employers, schools, and Federal, state, and local health departments across the country—Thermo Fisher Scientific, the CDC, the State of California and the Commonwealth of Massachusetts, Chicago Public Schools, to name a few—to provide critical access where people worked, played, learned, and worshiped. When antiviral treatment became available, Color ran three-quarters of the country’s state-level telehealth programs, with prescription delivery within 24 to 48 hours of symptom onset. Our population health work has since expanded to support other infectious disease areas. Color currently runs the State of California’s HIV PrEP telehealth access program.
Over the years, we have continued to invest in access—offering multilingual support, flexible care delivery models, hotlines for those without smartphones or computers, and more—further cementing our commitment to broad population health.
Learn about one of our community partnerships below.
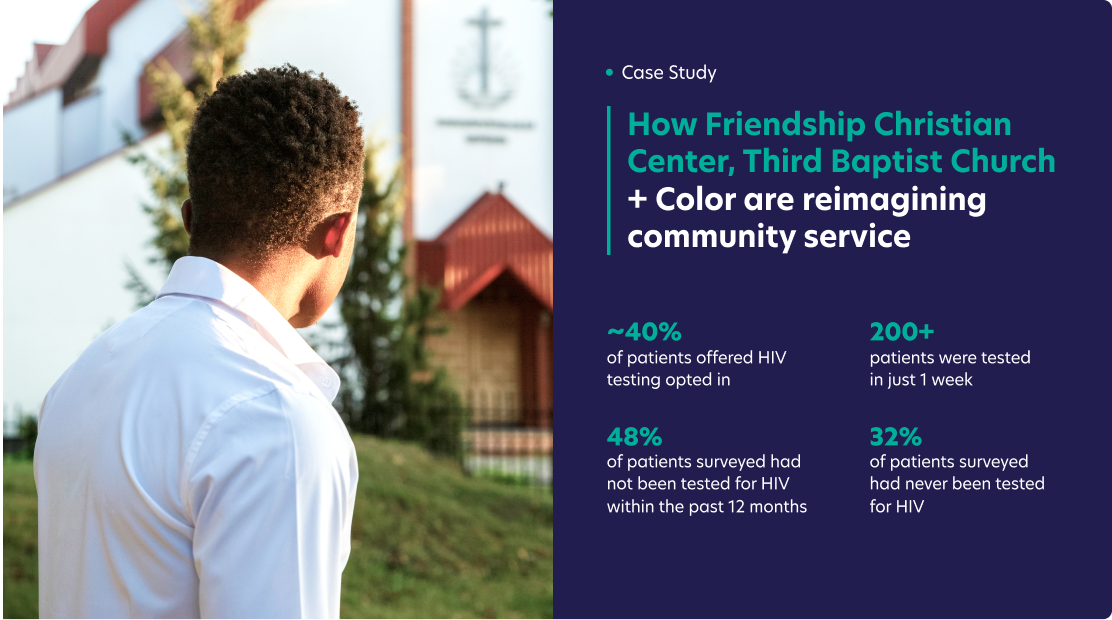
Color partners with the American Cancer Society to operationalize a joint mission of ending cancer as we know it.
In June 2023, Color and the American Cancer Society (ACS) introduced a cancer screening and prevention program for employers and labor organizations, combining the ACS’s expertise in cancer screening, prevention, and risk reduction with Color’s leadership in population-scale healthcare delivery.
Rates of cancer screening, stage of detection, and treatment outcomes reflect some of the deepest disparities in American healthcare. With 159 million Americans receiving healthcare coverage through their employer, the ACS and Color recognize the critical role employers play in removing barriers of access to timely and quality preventive care. Hear from Hasbro employee and cancer patient, Gina, on the importance of early detection and employer support for cancer patients and caregivers below.
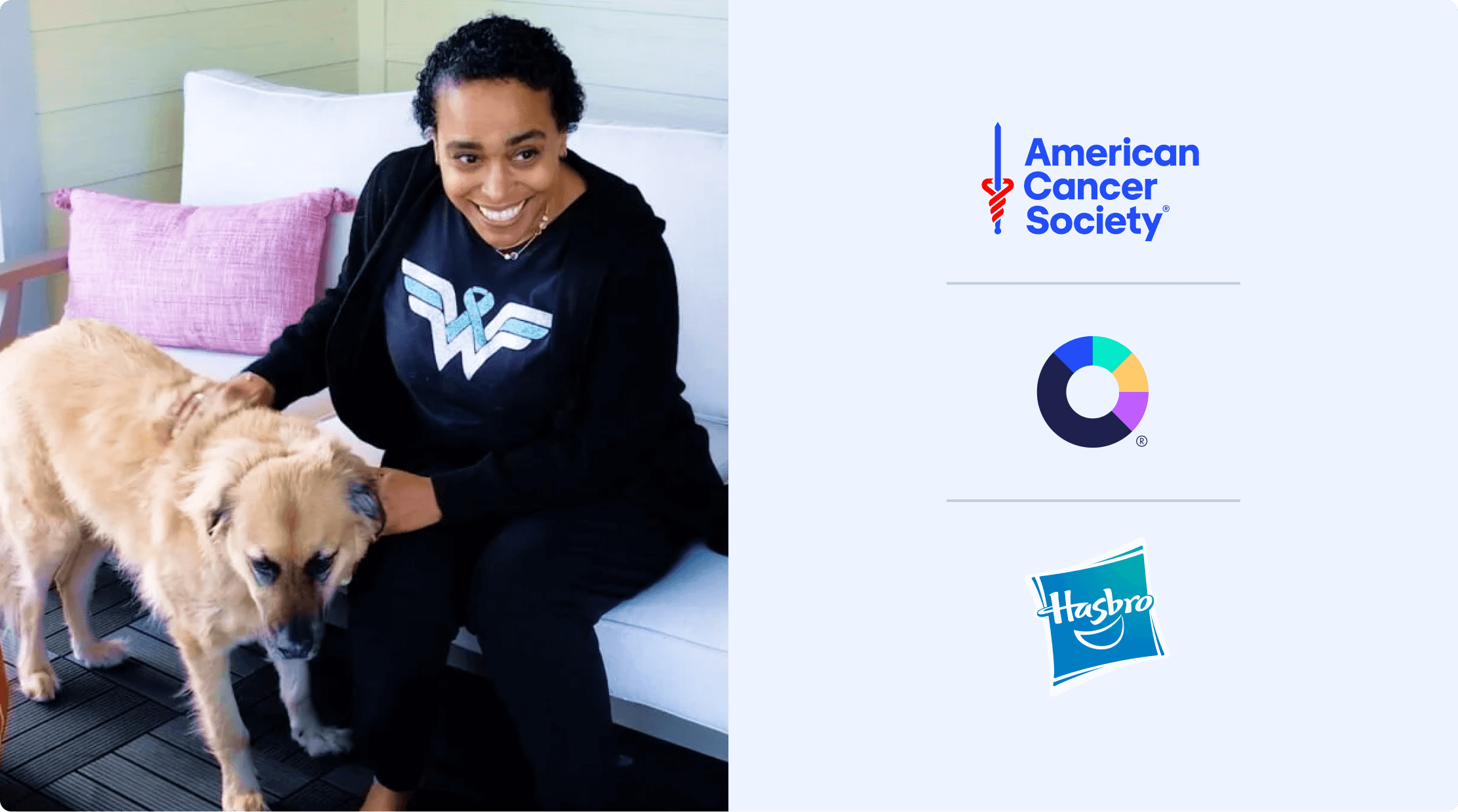
Color’s cancer care solution takes hold with employers, as the team joins forces with Hasbro, Shoestring Holdings, and more.
Today, our day-in, day-out focus is helping large purchasers take control of cancer. Since announcing our partnership with the American Cancer Society, our program has expanded to support diagnosis management, cancer treatment and care, and survivorship. Through our Virtual Cancer Clinic, Color is: helping employees and members diagnose cancer at an earlier, more treatable stage; caring for patients going through their diagnostic journeys with empathy and speed; helping cancer survivors navigate return-to-work challenges and complex clinical needs; and closing critical cancer screening gaps for high-burden cancers.
Seeing our first cohorts go live with the Color and American Cancer Society program has amplified our confidence that a comprehensive and integrated model will be the most effective way to change the survival and cost trajectory of cancer for millions of people across the United States.
In the continuing spirit of access, the White House recently highlighted our joint pledge with the American Cancer Society to provide free at-home colorectal cancer screenings for uninsured and under-insured individuals. Read the White House Fact Sheet below to learn more about the commitments made from other employers and industry leaders.
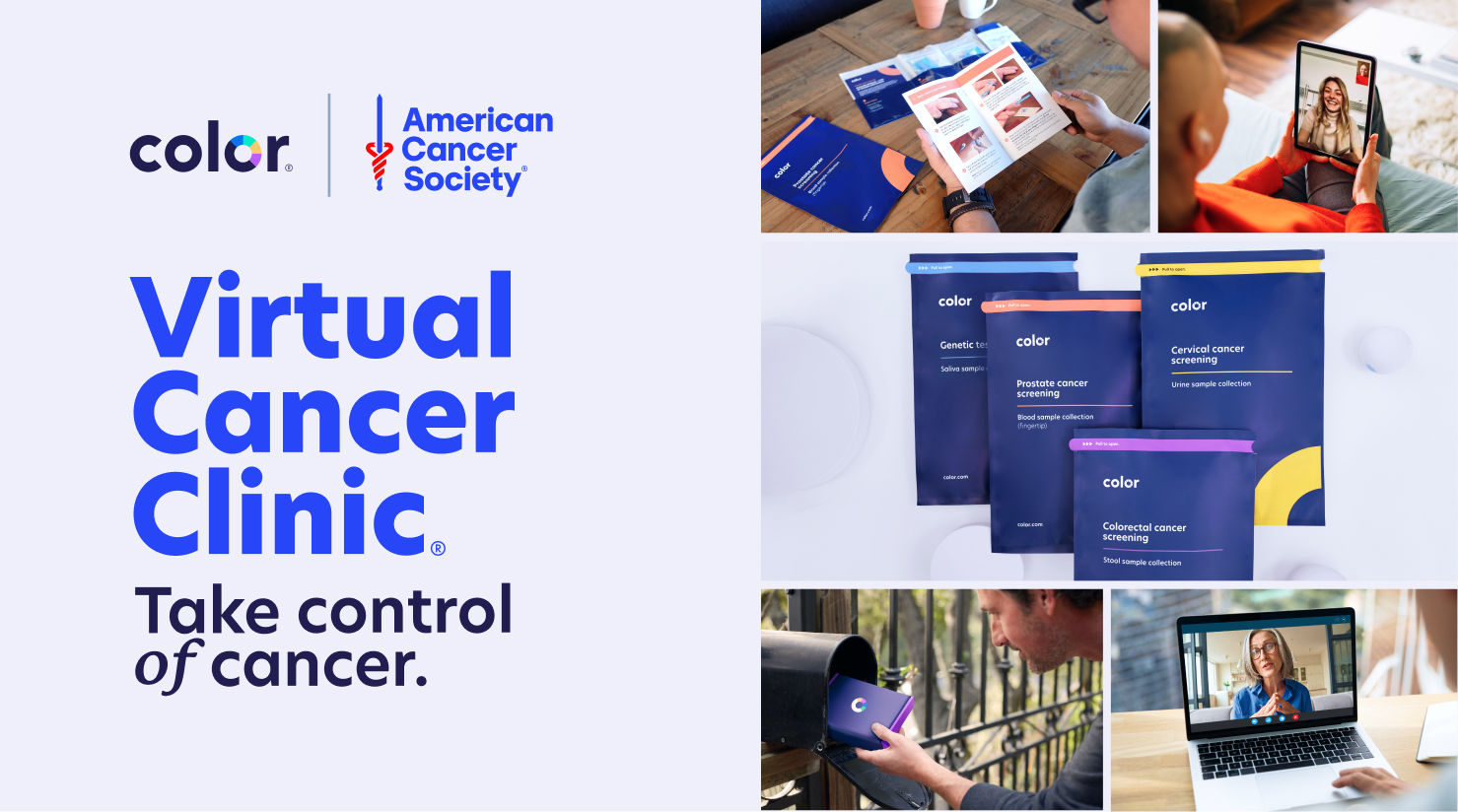
Color Health launches its first product to help make healthcare more affordable, accessible, and actionable.
In 2015, Color launched with a new clinical genetic testing model to help patients understand and take action on their personal risk of cancer. Our first product tested for BRCA1, BRCA2, and 17 other genes that increase an individual’s cancer risk. At the time, genetic tests for ovarian and breast cancer risk typically cost several thousand dollars, and access was often gated through long wait times for genetic counseling appointments at centers of excellence. Color was the first to deliver comprehensive genetic testing—including genetic counseling, at-home sample collection, and easy-to-understand reports—for less than a tenth of the cost. This affordable model has helped hundreds of thousands of individuals take action on their genetic risk.
Learn how Color worked with the Teamsters Health and Welfare Fund of Philadelphia and Vicinity to change how employees engaged with preventive care by better understanding their risk of cancer and other genetic diseases.
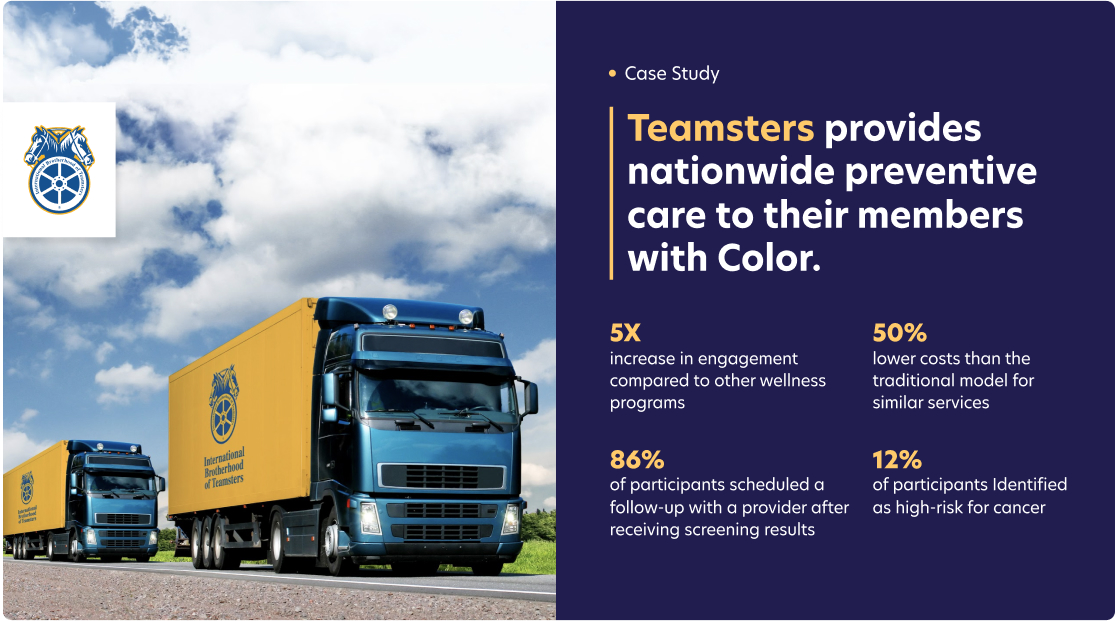
Color begins a long history of partnering with leaders in research and precision medicine, including the National Institutes of Health.
In 2018, the National Institutes of Health (NIH) awarded funding to Color, in partnership with the Broad Institute of MIT and Harvard, to establish one of three genome centers for its historic All of Us Research Program, a one million-participant program aimed at helping researchers better understand long-term health outcomes across the U.S. Color was initially selected to analyze and report genomic data for a set of 59 genes that cause conditions such as breast and ovarian cancer, hypercholesterolemia, Lynch syndrome, and more. Since then, Color has become the genetic counseling resource for all 1 million participants, the single home for test reporting, and the provider of ongoing participant engagement.
Since launching, the program has gathered data from hundreds of thousands of participants, over 80% of whom come from populations that have been historically underrepresented in clinical research. Our work with the NIH is the largest of our major research collaborations but not the last. We have since gone on to work with research institutions such as the Mayo Clinic, the Fred Hutchinson Cancer Research Center, and the University of Chicago and many local, state, and Federal governments.
Learn about an important NIH and Color partnership milestone—processing 100,000 genetic results for the All of Us Program—below.
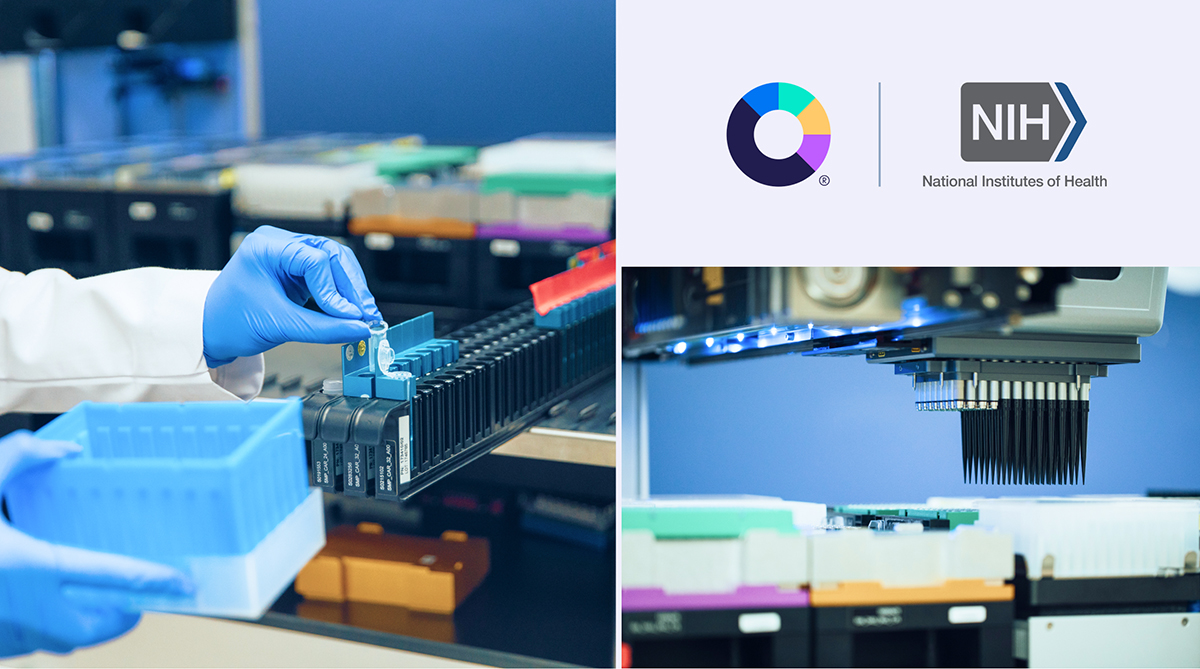
Color mobilizes to change access to critical COVID-19 testing, vaccination, and treatment services.
On March 16th, 2020, the first stay-at-home orders were issued in the San Francisco Bay Area. Exactly two weeks later, Color had built a CLIA-certified COVID-19 testing lab and digital platform. One week after that, Color and the City and County of San Francisco launched one of the first high-capacity, public COVID testing sites.
In the months and years to come, Color supported employers, schools, and Federal, state, and local health departments across the country—Thermo Fisher Scientific, the CDC, the State of California and the Commonwealth of Massachusetts, Chicago Public Schools, to name a few—to provide critical access where people worked, played, learned, and worshiped. When antiviral treatment became available, Color ran three-quarters of the country’s state-level telehealth programs, with prescription delivery within 24 to 48 hours of symptom onset. Our population health work has since expanded to support other infectious disease areas. Color currently runs the State of California’s HIV PrEP telehealth access program.
Over the years, we have continued to invest in access—offering multilingual support, flexible care delivery models, hotlines for those without smartphones or computers, and more—further cementing our commitment to broad population health.
Learn about one of our community partnerships below.
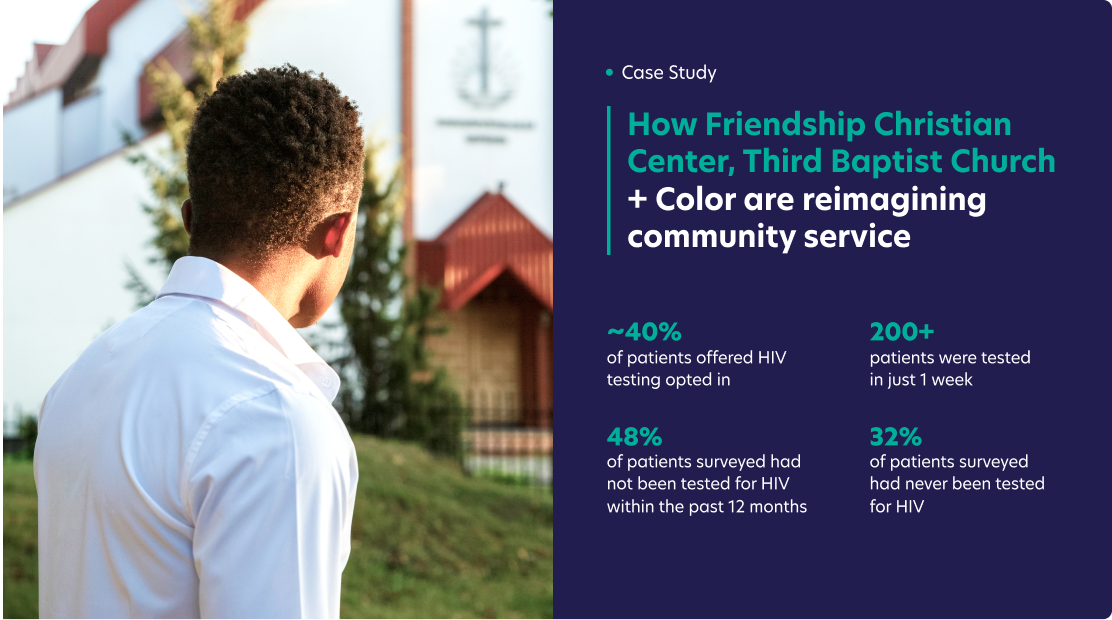
Color partners with the American Cancer Society to operationalize a joint mission of ending cancer as we know it.
In June 2023, Color and the American Cancer Society (ACS) introduced a cancer screening and prevention program for employers and labor organizations, combining the ACS’s expertise in cancer screening, prevention, and risk reduction with Color’s leadership in population-scale healthcare delivery.
Rates of cancer screening, stage of detection, and treatment outcomes reflect some of the deepest disparities in American healthcare. With 159 million Americans receiving healthcare coverage through their employer, the ACS and Color recognize the critical role employers play in removing barriers of access to timely and quality preventive care. Hear from Hasbro employee and cancer patient, Gina, on the importance of early detection and employer support for cancer patients and caregivers below.
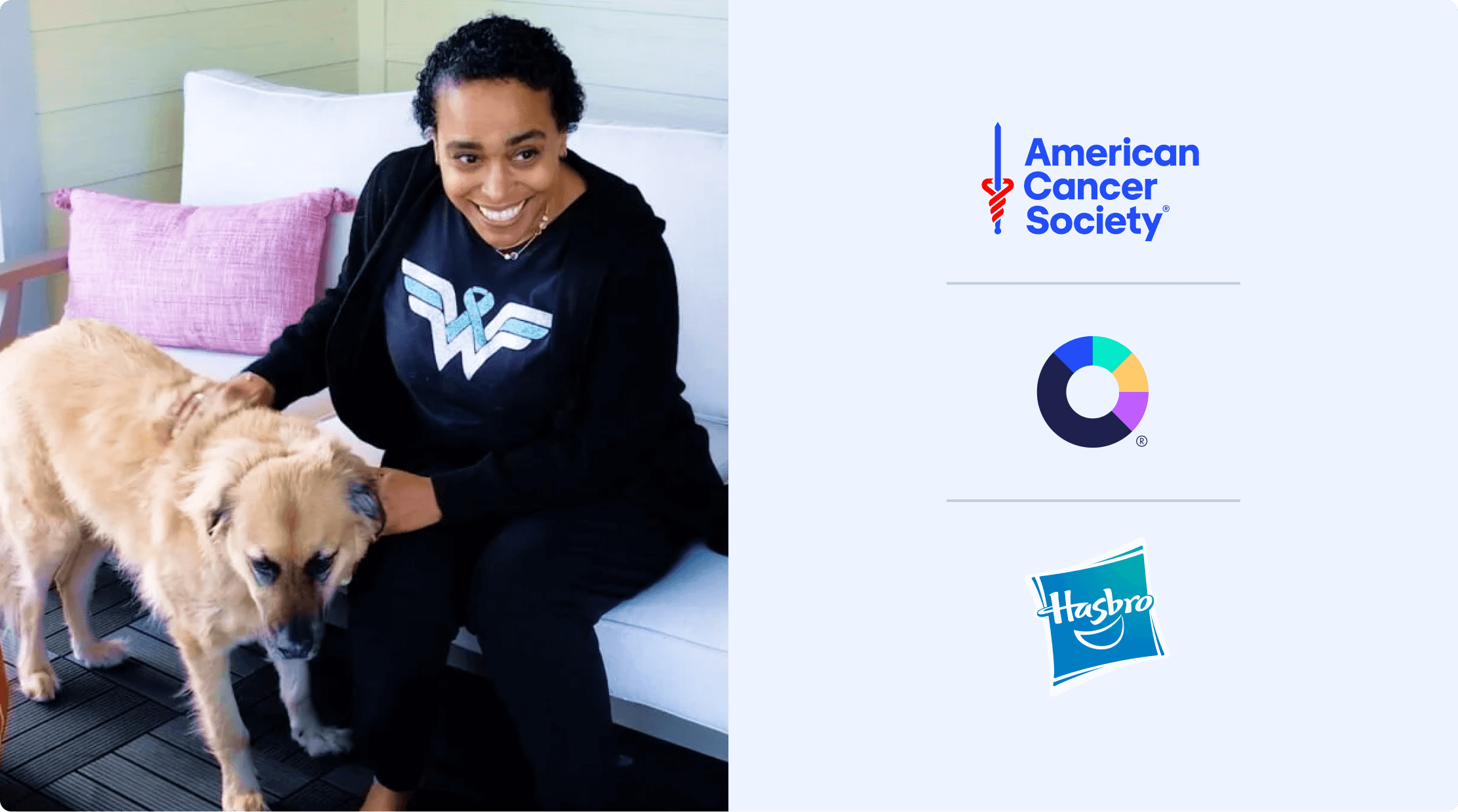
Color’s cancer care solution takes hold with employers, as the team joins forces with Hasbro, Shoestring Holdings, and more.
Today, our day-in, day-out focus is helping large purchasers take control of cancer. Since announcing our partnership with the American Cancer Society, our program has expanded to support diagnosis management, cancer treatment and care, and survivorship. Through our Virtual Cancer Clinic, Color is: helping employees and members diagnose cancer at an earlier, more treatable stage; caring for patients going through their diagnostic journeys with empathy and speed; helping cancer survivors navigate return-to-work challenges and complex clinical needs; and closing critical cancer screening gaps for high-burden cancers.
Seeing our first cohorts go live with the Color and American Cancer Society program has amplified our confidence that a comprehensive and integrated model will be the most effective way to change the survival and cost trajectory of cancer for millions of people across the United States.
In the continuing spirit of access, the White House recently highlighted our joint pledge with the American Cancer Society to provide free at-home colorectal cancer screenings for uninsured and under-insured individuals. Read the White House Fact Sheet below to learn more about the commitments made from other employers and industry leaders.
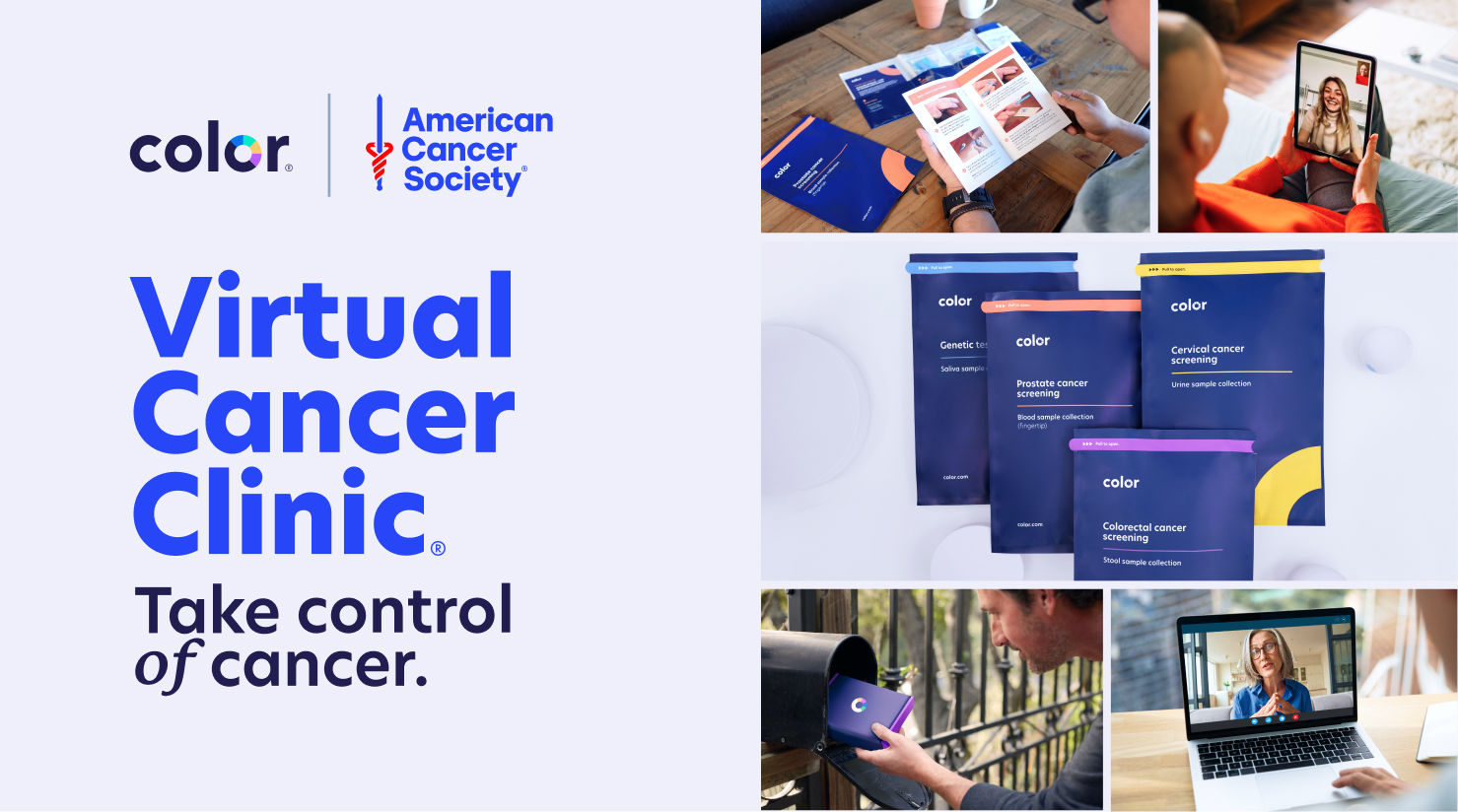
Our population-scale impact
>7M
patients served
>26M
healthcare interventions delivered
>13K
healthcare access sites
>2K
organizations supported
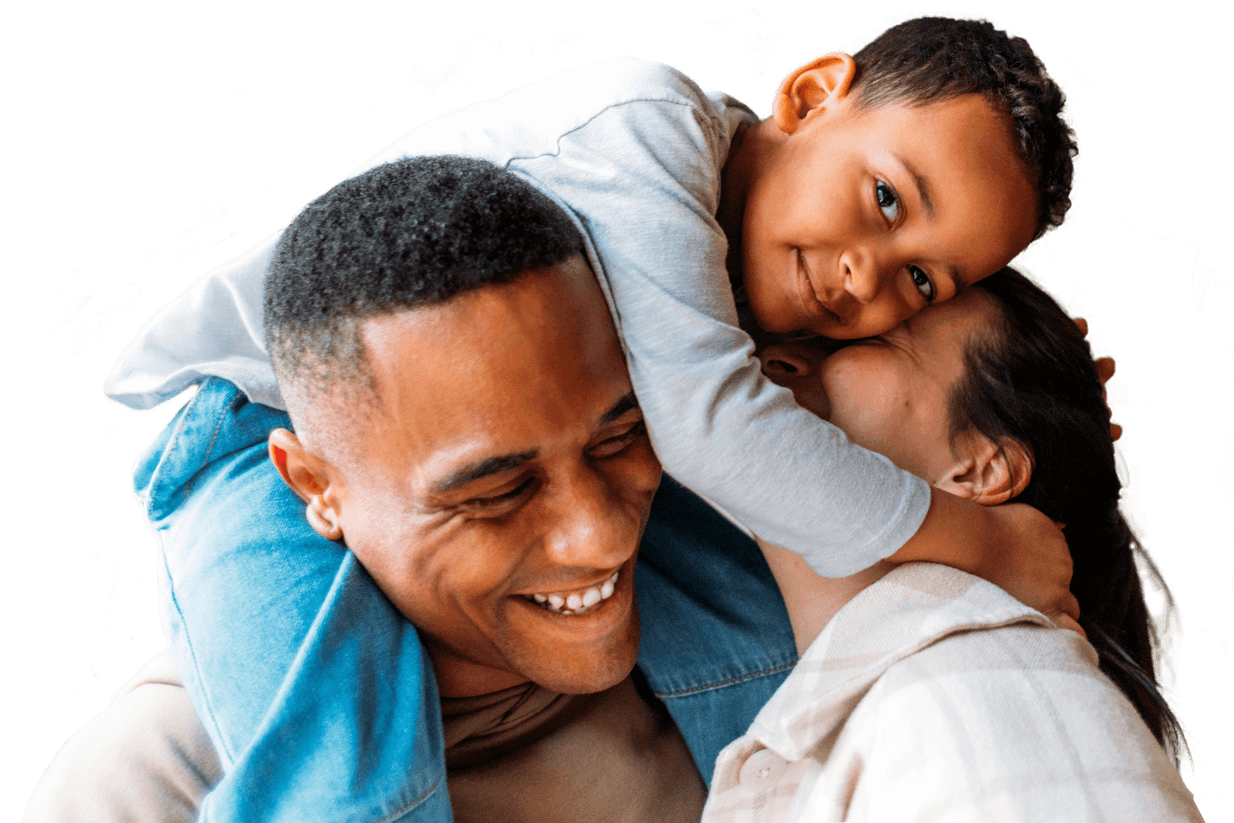
Our partnership with the American Cancer Society
Together, Color and American Cancer Society offer a comprehensive cancer solution for employers, health plans, and labor unions, with the goal of supporting their employee and member populations across all stages of the cancer journey. The solution combines the ACS’s expertise in cancer screening, prevention, and risk reduction with Color’s leadership in population-scale healthcare delivery, enabling organizations and individuals to a) detect cancer earlier and b) manage cancer more effectively.
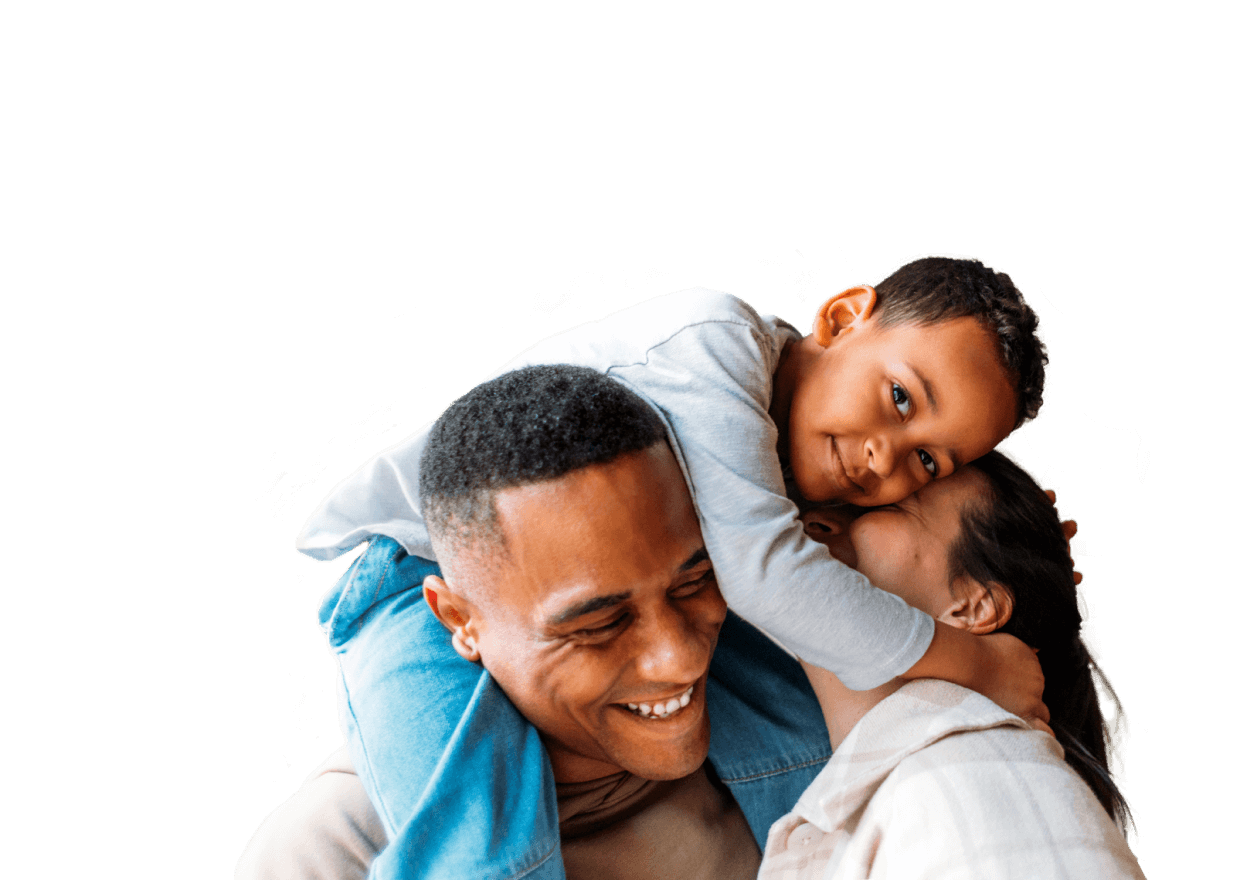
Our partnership with the American Cancer Society
Together, Color and American Cancer Society offer a comprehensive cancer solution for employers, health plans, and labor unions, with the goal of supporting their employee and member populations across all stages of the cancer journey. The solution combines the ACS’s expertise in cancer screening, prevention, and risk reduction with Color’s leadership in population-scale healthcare delivery, enabling organizations and individuals to a) detect cancer earlier and b) manage cancer more effectively.
“The biggest impact happens when we meet patients and their families where they live, work, and play. Comprehensive support for cancer can start in the workplace. At the American Cancer Society, we’re proud to work with Color to help employers take action.”
At Color, we remove the logistical, technological, financial, and cultural barriers that accompany traditional healthcare delivery. With a decade of experience in the cancer and high-risk space, Color has served millions of patients by partnering with innovative organizations that prioritize the health of their workforces and populations.
Our technology and clinical care models are scalable but patient-centered—built with infrastructure that meets the needs of large organizations, but designed for ease, availability, and accessibility by each individual patient.
Trusted by leading organizations in every industry
Let’s work together to take control of cancer.
Are you interested in shaping the future of healthcare? Are you passionate about applying engineering, design, science, and other technological and creative disciplines to improve people’s health and quality of life on a global scale? If so, we want to work with you.
Our laboratory licensing and accreditation
Color has an advanced, CAP-accredited and CLIA-certified laboratory that uses the newest technology, such as acoustic liquid handlers and autonomous, self-optimizing robotic systems, to ensure the integrity of every sample.
Color’s licenses and certifications include:
CAP
8975161
CLIA
05D2081492
California
CDF 00346276
New York
PFI 9069
Maryland
2376
Pennsylvania
034727
Rhode Island
LCO01577
District of Columbia
HFD-OS38
Speak with our solutions team.
Want to get in touch with our team? We’re just a click away.
New to Color?
Speak to our team to discuss your cancer strategy and Color’s solution.
Already a patient?
If you need help with your account, have questions for your care team, or have other service-related issues, we’re here.
New to Color?
Whether you want to learn more about Color or you’re ready to start your journey with us, you’re in the right place.
Already a customer? Get support.
Call us toll-free between 6am and 5pm PT at (844) 352-6567. Those outside the US can reach us at +1 650-651-7116. We’re also available via email at support@color.com.
For frequently asked questions please visit support.color.com.